Data Analysts are not Junior Data Scientists
Mar 30
/
Sujal Dhungana
Data Analyst and Data Scientist are two distinct roles that require a separate set of skills, although both deal with data to generate insights. The tools they use and the perspectives they apply to problems differ significantly. Contrary to popular belief, the data scientist role is not necessarily a career progression or "dream role" for a data analyst. Both data analysts and data scientists have their unique career trajectories. You can grow your Data analyst career as much as you can imagine without entering the data science realm and vice versa.
Data Analyst
A data analyst's primary focus is on collecting, organizing, and analysing data to provide actionable insights that inform business decisions. They often work with structured data sources, such as databases and spreadsheets, and use tools like SQL, Excel, and data visualization software (e.g., Tableau, Power BI) to extract, clean, and present data in a meaningful way.
Tools
-
Excel
-
SQL
-
Power BI
-
Tableau
Data Scientist
A data scientist's role is more technical and involves developing and applying advanced statistical and machine learning models to uncover insights and make predictions from complex, often unstructured data sources. They typically have a strong background in mathematics, statistics, and programming languages like Python and R.
Tools
-
Python
-
R
-
Statistics
-
Machine Learning
Imaginary Retail Company
Let's imagine a fictional retail company which employes both Data Analyst and Data Scientist.
Data Analysts in this company will focus on analysing sales data to identify trends, customer buying patterns, and factors that influence purchasing decisions. They would use SQL to query the sales database, clean and manipulate the data in Excel, and then create visualizations in Tableau to communicate their findings to stakeholders.
Data Scientist might develop predictive models to forecast sales trends, create customer segmentation for targeted marketing, and use machine learning for personalized product recommendations. They could also employ natural language processing for sentiment analysis of customer feedback and build algorithms for price optimization and fraud detection.
In this example, their responsibilities and focus are different but together they are working towards a common objective which is to generate value for the company.
Data Analysts in this company will focus on analysing sales data to identify trends, customer buying patterns, and factors that influence purchasing decisions. They would use SQL to query the sales database, clean and manipulate the data in Excel, and then create visualizations in Tableau to communicate their findings to stakeholders.
Data Scientist might develop predictive models to forecast sales trends, create customer segmentation for targeted marketing, and use machine learning for personalized product recommendations. They could also employ natural language processing for sentiment analysis of customer feedback and build algorithms for price optimization and fraud detection.
In this example, their responsibilities and focus are different but together they are working towards a common objective which is to generate value for the company.
The comparison
While both roles involve working with data, the skills and responsibilities differ significantly.
Data Analysts primarily focus on descriptive and diagnostic analytics, answering questions like "What happened?" and "Why did it happen?" They provide insights that support decision-making processes. Data Analysts will work more closely with the business to understand the problem and identify relevant data required to either understand the problem better or devise data-driven solutions.
Data Analysts primarily focus on descriptive and diagnostic analytics, answering questions like "What happened?" and "Why did it happen?" They provide insights that support decision-making processes. Data Analysts will work more closely with the business to understand the problem and identify relevant data required to either understand the problem better or devise data-driven solutions.
Data Scientists delve into predictive and prescriptive analytics, addressing questions like "What will happen?" and "What should we do?" They develop advanced models and algorithms to forecast future trends and prescribe optimal solutions. Essentially, the Data Scientist would delve into predictive and prescriptive analytics, using complex models to not only interpret data but to anticipate future trends and guide strategic decisions.
It's important to note that neither role is inherently superior or a natural progression from the other. Both data analysts and data scientists play crucial roles in organizations, and their careers can progress within their respective domains.
Career Progression
Data Analysts may advance to roles like senior data analyst, data analytics manager, or business intelligence analyst, where they oversee more complex data projects, manage teams, and work closely with stakeholders to drive strategic decisions.
Similarly, Data Scientists can progress to roles such as lead data scientist, machine learning engineer, or research scientist, where they develop cutting-edge algorithms, lead data science teams, and contribute to the advancement of their field through research and innovation.
Having said that anyone willing to switch career from either focus area can easily do so. They have to upskill themselves in some of the tools if they are not already familiar with or the necessary soft skills if the position requires so.
Having said that anyone willing to switch career from either focus area can easily do so. They have to upskill themselves in some of the tools if they are not already familiar with or the necessary soft skills if the position requires so.
Conclusion
Knowing the distinctions between various data careers is particularly important when you're considering a path in this field. Understanding the differences between these roles is crucial for individuals considering careers in data-related fields and for organizations seeking to leverage the unique strengths of each role effectively. A lack of understanding about the specific roles and the skills they require can lead to confusion and a lack of direction in terms of what to learn and which positions to aim for.
Here's a bit of guidance to help you: Find what excites you.
If your passion lies in collaborating with businesses to pinpoint issues, presenting data insights to stakeholders, creating visualizations, and crafting compelling data narratives, then a career as a Data Analyst might be your calling. Conversely, if you're drawn to diving deeply into data, exploring the realms of mathematics and statistics, and developing advanced models to tackle complex issues, then the field of Data Science might be the right fit for you.
Want more tailored help? Reach out to us for a free 30-minute career counselling where our expert career mentors will listen to your aspirations and build a customised development plan just for you.
Here's a bit of guidance to help you: Find what excites you.
If your passion lies in collaborating with businesses to pinpoint issues, presenting data insights to stakeholders, creating visualizations, and crafting compelling data narratives, then a career as a Data Analyst might be your calling. Conversely, if you're drawn to diving deeply into data, exploring the realms of mathematics and statistics, and developing advanced models to tackle complex issues, then the field of Data Science might be the right fit for you.
Want more tailored help? Reach out to us for a free 30-minute career counselling where our expert career mentors will listen to your aspirations and build a customised development plan just for you.
Latest from our blog
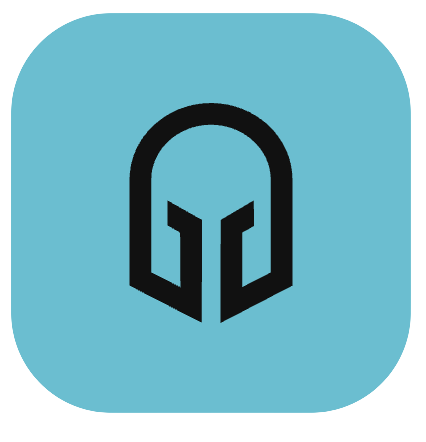
We at Data Professional Academy are dedicated to nurturing the next generation of data professionals. We are the only learning platform that guarantees career support until you land your first data job. So if you want to land your first data job, join us today.
Copyright © 2024 Data Professional Academy | ABN 86 670 807 333
FEATURED LINKS
CONNECT WITH US
-
Facebook
-
Twitter
-
YouTube
-
Instagram
-
LinkedIn